Transforming Agriculture with Machine Learning: The Impact of Datasets
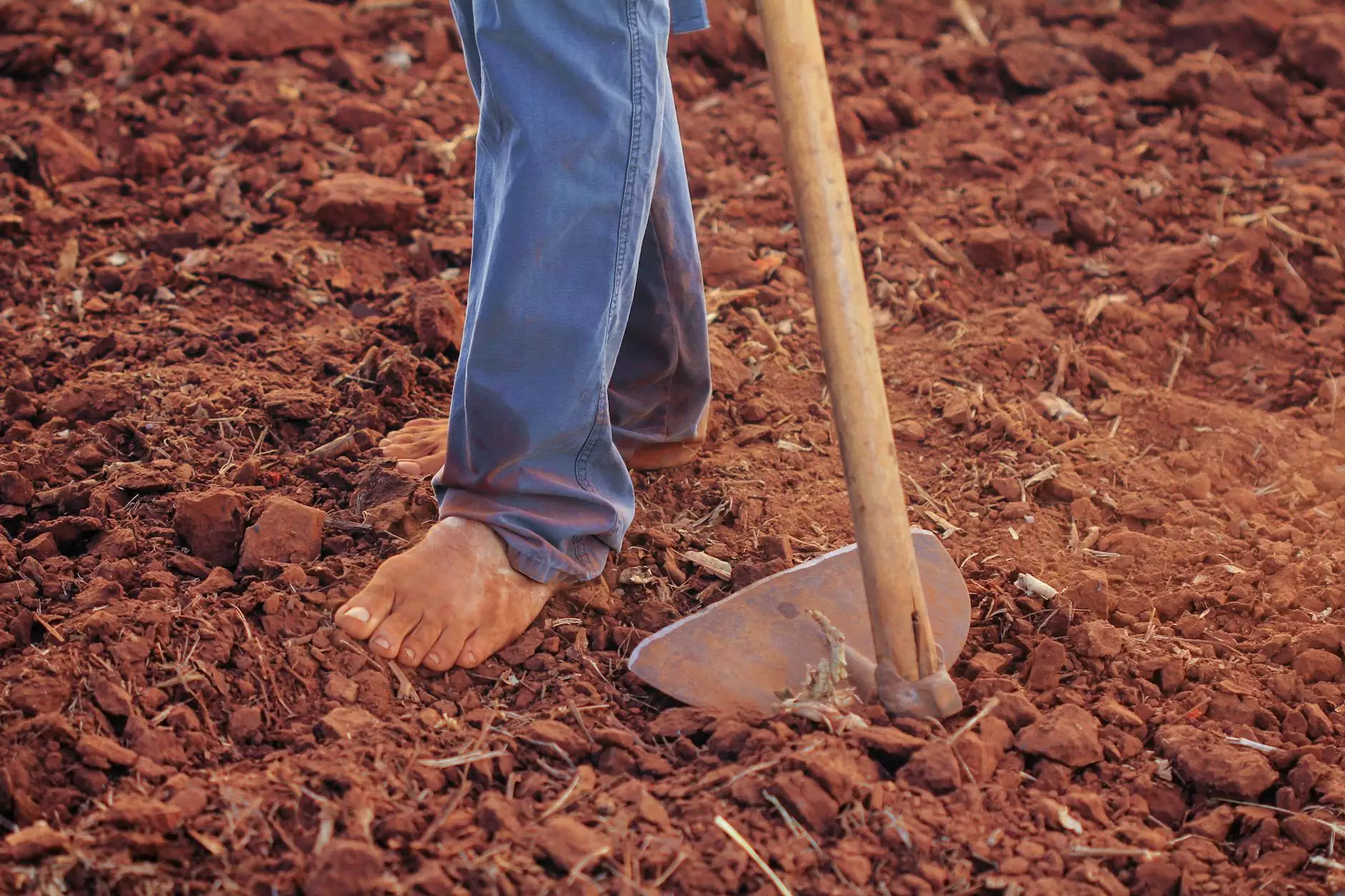
In recent years, the agricultural sector has experienced a significant transformation due to the integration of innovative technologies. One of the key drivers of this transformation is the use of machine learning, which leverages vast amounts of data to enhance decision-making and operational efficiency. Central to the success of machine learning in agriculture is the availability of high-quality agriculture dataset for machine learning. This article delves into the importance of these datasets, their applications, and the future of agriculture as shaped by machine learning technologies.
The Importance of Agriculture Datasets
Datasets in agriculture are collections of data that can include variables such as crop yields, weather patterns, soil moisture, and more. These datasets are crucial for developing machine learning models that can:
- Predict Crop Yields: By analyzing historical data, machine learning algorithms can forecast crop yields based on various factors.
- Optimize Resource Use: Datasets can help in creating models that optimize water usage, fertilizers, and pesticides, reducing waste and costs.
- Improve Pest Management: Understanding patterns in pest populations allows for timely interventions, reducing crop loss.
- Enhance Supply Chain Efficiency: Data-driven approaches can improve logistics from farm to table.
Types of Agriculture Datasets
When it comes to the agriculture dataset for machine learning, it is essential to understand the different types of data available:
1. Soil Data
This includes pH levels, nutrient content, and moisture levels. Soil data can help predict productivity and inform decisions on crop selection and land management.
2. Weather Data
Weather data encompasses temperature, precipitation, and humidity levels over time. This information is vital for crop planning and risk assessment.
3. Yield Data
Historical yield data provides insights into the expected productivity of different crops under varying conditions.
4. Market Data
Market datasets include pricing trends and consumer demand analytics, which can greatly influence planting decisions and market strategies.
Machine Learning Applications in Agriculture
Machine learning applications in agriculture are vast and varied. Here are some key areas where these technologies are making a significant impact:
1. Precision Farming
Precision farming utilizes machine learning algorithms to analyze data collected from sensors, drones, and satellites to optimize field-level management. This results in better yields, reduced input costs, and decreased environmental impact.
2. Crop Monitoring
Farmers can employ machine learning models to monitor crop health through remote sensing data. This technology allows for early detection of diseases and helps in taking timely actions to minimize losses.
3. Automated Irrigation Systems
With the help of machine learning, automated irrigation systems can adjust water supply based on real-time data about soil moisture and weather forecasts, promoting sustainable water use.
The Future of Agriculture and Technology
The future of agriculture is poised to be exceedingly exciting as machine learning continues to evolve. Here’s how:
1. Data Integration
As more devices in agriculture become connected (IoT), the amount of data available will grow exponentially. Integrating diverse datasets can lead to more comprehensive models that can predict outcomes with greater accuracy.
2. Smart Farming Techniques
Technologies such as automated tractors and drones will become smarter, powered by robust machine learning models that ensure better efficiency and productivity.
3. Sustainability Goals
Machine learning can significantly aid in achieving sustainability goals by optimizing resource use and minimizing waste, thus contributing to more sustainable food production systems.
The Challenges Ahead
Despite the benefits, there are challenges in utilizing agriculture datasets for machine learning. These challenges include:
- Data Quality: The effectiveness of machine learning models is heavily reliant on the quality of the datasets.
- Access to Data: Small-scale farmers may not have access to the necessary data infrastructure.
- Skill Gap: The gap in knowledge related to data analysis and machine learning can hinder adoption.
Conclusion: Embracing Innovation in Agriculture
In conclusion, the integration of machine learning into agriculture represents a significant advancement in how we approach farming. The reliance on high-quality agriculture dataset for machine learning cannot be understated, as it is vital for training models that drive informed decisions in the agricultural sector. As technology continues to evolve, the opportunities for enhancing productivity, sustainability, and efficiency in agriculture will only grow, paving the way for a new era in food production.
For those in the agricultural business, embracing these changes and investing in the right technologies will be crucial for staying competitive in an ever-evolving industry. As we continue to navigate these changes, it is essential to foster a culture of innovation and adaptability.
Resources for Further Learning
If you're interested in exploring more about the intersection of agriculture and machine learning, here are some resources to consider:
- TechTarget: Agriculture Analytics
- ScienceDirect: Machine Learning in Agriculture
- IBM: Machine Learning and IoT in Agriculture
- Forbes: How AI and Machine Learning Are Revolutionizing Agriculture