Unlocking the Power of Labeling Training Data for Business Success
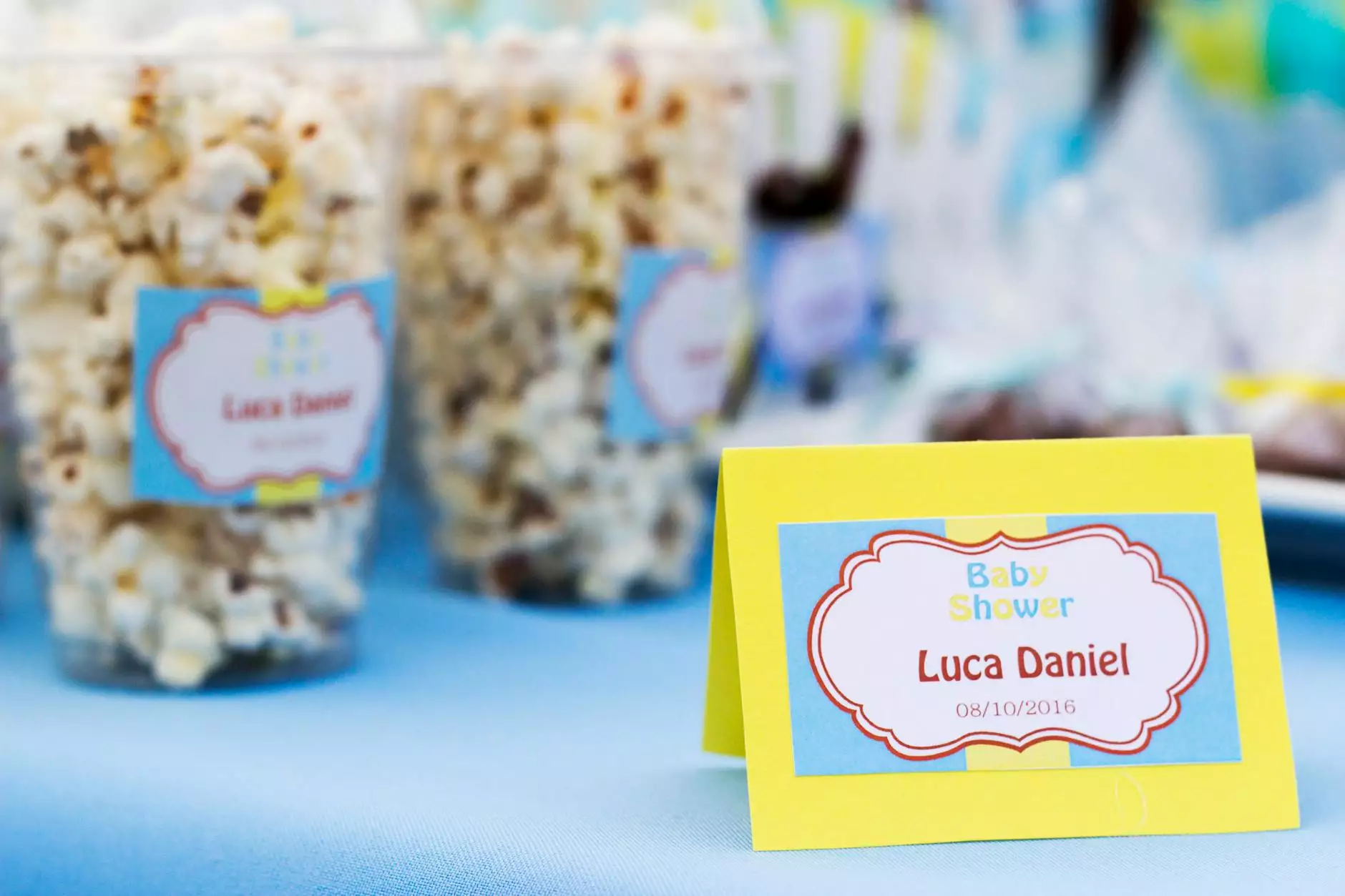
In today’s technology-driven world, the success of any software application largely depends on the quality of the labeling training data used to train its underlying machine learning algorithms. As businesses pivot towards utilizing artificial intelligence (AI) and machine learning (ML), understanding the intricacies of data labeling is essential for staying competitive in the market. In this comprehensive article, we will explore the significance of labeling training data, effective strategies to implement it, and how it can lead to substantial business growth alongside improved software development practices.
The Foundations of Machine Learning: Importance of Training Data
Machine learning is fundamentally reliant on data. The algorithms learn from the data fed into them, making the quality and accuracy of that data paramount. Without high-quality training data, even the most sophisticated algorithms can fail to deliver optimal results. Here are the key reasons why labeling training data is an integral part of the software development process:
- Enhances Model Accuracy: Properly labeled data directly influences the accuracy of predictions made by machine learning models. Well-defined labels help algorithms distinguish between different classes or categories, leading to better performance in real-world applications.
- Facilitates Comprehensive Learning: By providing clear and consistent labels, businesses can ensure that models learn all relevant features of the data. This comprehensiveness allows for more nuanced understanding and adaptability to new information.
- Reduces Bias: Accurate labeling helps in identifying and mitigating biases present within datasets. By ensuring diverse and representative labeling, businesses can develop models that serve a broader audience without favoring specific groups.
- Improves Model Interpretability: Transparent and precise labeling aids in understanding how algorithms arrive at their decisions. This is particularly important in sectors where ethical considerations and accountability are vital.
Strategic Approaches to Labeling Training Data
To maximize the effectiveness of labeling training data, organizations must adopt strategic approaches. Here are several methods that can significantly enhance the labeling process:
1. Define Clear Labeling Guidelines
Establishing well-defined labeling criteria is crucial. These guidelines should provide clear instructions regarding what each label represents and how it should be applied across different datasets. This ensures consistency and reduces the risk of ambiguities that can lead to misrepresentations.
2. Utilize Advanced Labeling Tools
The market offers various advanced labeling tools that can simplify the data labeling process. These tools often come with features such as:
- Automated labeling suggestions
- Collaboration features for team-based labeling efforts
- Quality control measures to ensure accuracy and consistency
Employing such tools can save time while improving the quality of labeled data.
3. Engage Domain Experts
Collaborating with individuals who possess domain-specific knowledge can greatly enhance the quality of labeling. Experts can provide insights into the context and nuances that non-experts might overlook, leading to more accurate and meaningful labels.
4. Iterative Labeling and Feedback Loops
Labeling should not be a one-off activity. Implementing iterative processes where labeled data is frequently reviewed and updated can help refine the quality of the dataset. Feedback loops allow teams to assess model performance and make necessary adjustments to labels.
Case Studies: Businesses Thriving Through Effective Data Labeling
To illustrate the power of labeling training data in driving business success, we will review several case studies of companies that have excelled by effectively managing their data labeling processes.
1. HealthTech Innovations
A leading HealthTech company utilized labeled training data to develop a predictive analytics tool for patient diagnosis. By employing domain experts to create precise labels related to medical conditions, symptoms, and treatment outcomes, they achieved a 95% accuracy rate in their predictive models. This accuracy directly translated into improved patient care and significant cost savings for healthcare providers.
2. E-Commerce Personalization
An e-commerce giant implemented advanced labeling techniques to categorize products based on customer behavior and preferences. By employing machine learning models trained on accurately labeled datasets, they achieved a 30% increase in sales through personalized recommendations, showcasing the impact of effective data labeling on their bottom line.
3. Autonomous Vehicles
In the realm of autonomous vehicles, a leading automotive manufacturer invested heavily in labeling training data to teach their models to identify various objects on the road. This included not just vehicles, but also pedestrians, traffic signals, and road conditions. Their commitment to accurate labeling accelerated their vehicle development processes and positioned them as pioneers in the self-driving technology landscape.
Challenges in Labeling Training Data
While labeling training data is essential for success, the process can come with challenges. Understanding these challenges is crucial for developing effective solutions:
1. Scalability Issues
As organizations grow, the volume of data can become overwhelming. Scaling up the labeling process without compromising quality can be difficult. Implementing automation and leveraging crowd-sourcing can alleviate some of these pressures.
2. Labeling Costs
The cost associated with high-quality data labeling can be significant. Businesses must balance the need for quality with their budget constraints. Outsourcing labeling tasks to specialized firms can often provide a cost-effective solution.
3. Ensuring Consistency
Maintaining consistent labeling across large datasets can be challenging, especially when multiple teams are involved. Regular training sessions and the use of automated tools can help in overcoming this challenge.
Future Trends in Data Labeling
The landscape of labeling training data is constantly evolving, driven by advancements in technology and changing business needs. Here are some trends that are shaping the future:
1. Increased Automation
Automation in data labeling is on the rise. With the integration of AI tools that can suggest labels based on existing data patterns, the manual effort required is decreasing. This evolution will allow organizations to label data with greater speed and accuracy.
2. Crowd-sourcing and Collaborative Labeling
Crowd-sourcing enables businesses to tap into a wider pool of labeling resources, making it easier to obtain diverse and representative labels. This collaborative approach can enhance both the quality and richness of the training data.
3. Real-time Data Labeling
With the growth of real-time applications, there is a trend towards real-time data labeling where data is labeled on-the-fly as it is generated. This is particularly useful in dynamic environments such as social media analysis or online customer interactions.
Conclusion: The Business Imperative for Labeling Training Data
As businesses navigate the complexities of the digital age, the importance of labeling training data cannot be overstated. With a well-structured approach to data labeling, organizations can unlock the full potential of their machine learning models, leading to enhanced accuracy, reduced biases, and ultimately, improved business outcomes.
Investing in quality labeling processes is not just a technical necessity; it is a strategic advantage in a competitive market. By prioritizing data labeling, businesses like keymakr.com can align their technological capabilities with market demands, driving innovation and ensuring sustained growth.