The Importance of Labelling Tools in Machine Learning
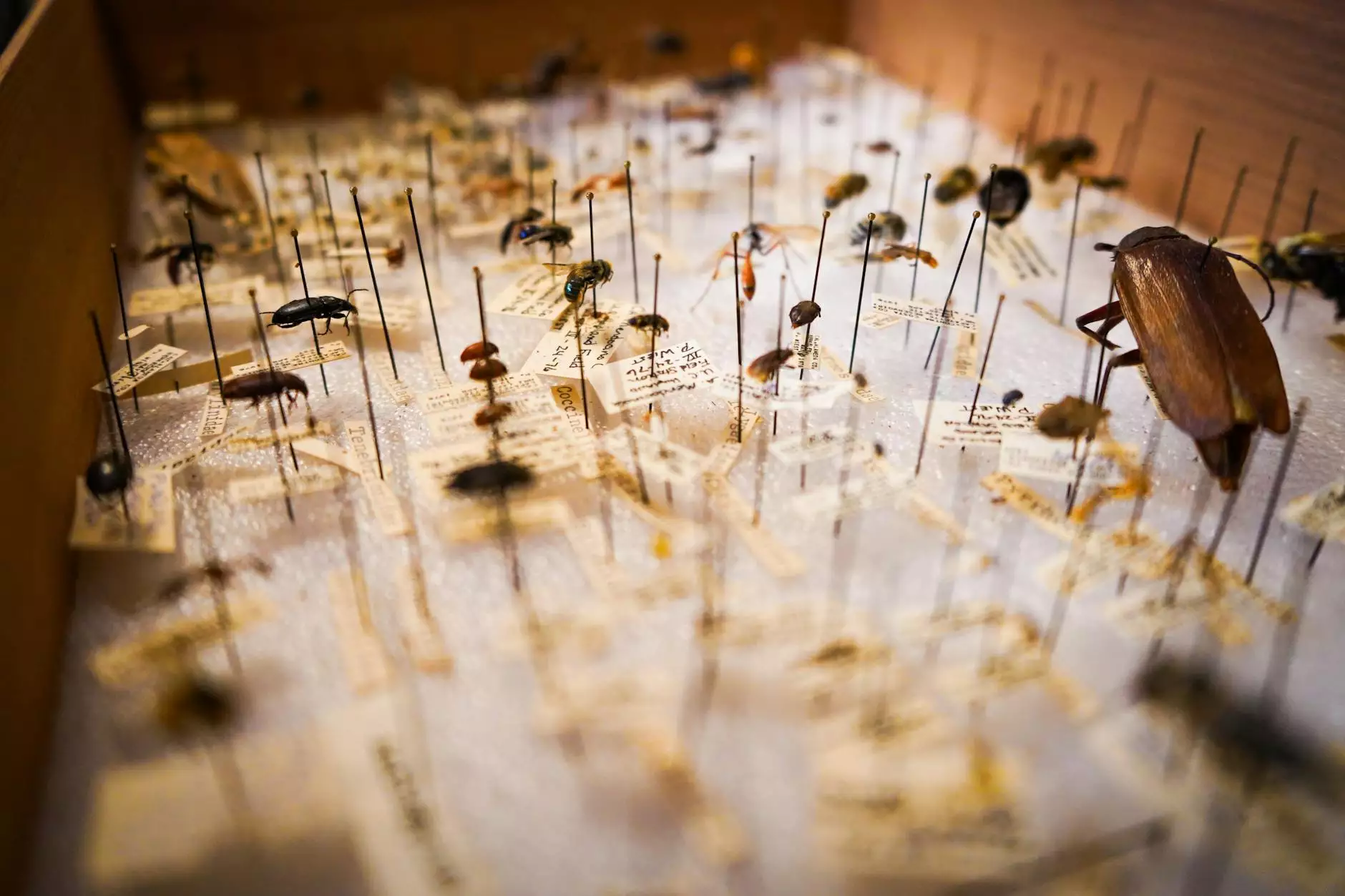
Introduction to Labelling Tools
In the realm of machine learning, data acts as the core foundation upon which models are built and predictions are made. One of the crucial steps in preparing this data is through effective labelling tools. These tools streamline the process of assigning labels or tags to data, which is essential for training models in a supervised learning environment. At KeyLabs.ai, our data annotation platform offers advanced labelling solutions that cater to various business needs.
The Role of Labelling Tools in Data Annotation
The process of data annotation is increasingly becoming pivotal in machine learning projects. Labelling tools are designed to facilitate this process by providing intuitive interfaces and automated features that allow for:
- Efficiency: Speeding up the labelling process significantly.
- Accuracy: Reducing human error through smart suggestions and automated functionalities.
- Scalability: Enabling businesses to scale their data annotation efforts without a decline in quality.
These characteristics make labelling tools indispensable in the toolkit of data scientists and machine learning engineers.
Understanding Machine Learning Models
Machine learning models learn from labelled data to make accurate predictions. Without proper labelling, models can misinterpret data, leading to poor outcomes. The types of machine learning algorithms that benefit from effective labelling include:
- Classification algorithms: Require distinct categories for each data point.
- Regression algorithms: Benefit from precise numeric labelling.
- Object detection and segmentation: Thrive on detailed pixel-level labelling in images.
Understanding these applications highlights the essential role that labelling tools play in the integrity of machine learning systems.
Key Features of Effective Labelling Tools
When selecting a labelling tool for your machine learning projects, consider the following features that enhance the data annotation process:
- Intuitive User Interface: A user-friendly design that minimizes the learning curve for annotators.
- Support for Various Data Types: Compatibility with images, text, audio, and video data.
- Collaboration Features: Tools that allow teams to work together seamlessly, tracking changes and updates in real time.
- Automated Labelling Options: Machine learning algorithms that assist in pre-labelling can save time and effort.
- Quality Control Measures: Built-in verification processes to ensure the accuracy of labels.
By focusing on these features, businesses can ensure they select the best labelling tool for their machine learning demands.
The Advantages of Automation in Labelling
Today's labelling tools increasingly integrate automation, revolutionizing the data annotation landscape. Here’s how automation delivers advantages:
- Increased Throughput: Automated processes can label data at a scale and speed unattainable by human annotators alone.
- Consistency: Automated labelling ensures uniformity across data sets, critical for effective training.
- Cost Efficiency: Reducing manual labour requirements translates to lower operational costs.
When selecting a labelling tool, ensure it incorporates these automated features to maximize your machine learning project’s potential.
Data Quality and Its Implications for Machine Learning
Data quality is paramount in machine learning, as high-quality, well-labelled data directly influences model performance. Poor quality data leads to:
- Inaccurate Predictions: Models trained on poor-quality data can make flawed predictions.
- Increased Costs: Time and resources wasted on cleaning and re-annotating data can be substantial.
- Loss of Trust: Stakeholders lose confidence in systems that produce unreliable results.
Utilizing top-tier labelling tools is critical to uphold data quality and create robust machine learning models capable of delivering consistent results.
Best Practices for Data Annotation Using Labelling Tools
Successful data annotation via labelling tools involves adhering to several best practices:
- Set Clear Guidelines: Define specific criteria for labelling to maintain consistency.
- Train Your Annotators: Ensure that everyone understands the labelling process and guidelines.
- Employ a Review Process: Implement peer reviews to catch errors early.
- Utilize Feedback Loop: Gather feedback from model evaluations to refine labelling strategies.
- Monitor Data Quality: Regular checks to ensure ongoing data quality throughout the project lifecycle.
By following these practices, businesses can optimize their data annotation workflows, creating more reliable machine learning outcomes.
The Future of Labelling Tools in Machine Learning
The landscape of machine learning is rapidly evolving, and so are the tools that support it. The future of labelling tools includes:
- Enhanced AI Integration: Expect deeper integrations with AI, aiding in smarter labelling processes.
- Real-time Collaboration: Advanced collaborative tools that facilitate teamwork across distances.
- Adaptive Learning Systems: Tools that learn from the annotators to improve their suggestions over time.
- More Robust Data Security: As data privacy becomes increasingly important, expect labelling tools to enhance their security measures.
The continuous advancement in labelling tools marks an exciting future for machine learning, promising enhanced efficiency and quality.
Conclusion
In summary, the adoption of effective labelling tools in machine learning is crucial for any organization looking to harness the power of data. As we have explored, the benefits of these tools extend beyond just data annotation; they forge pathways to higher quality data, improved model performance, and ultimately, better business decisions. At KeyLabs.ai, we are committed to providing comprehensive data annotation solutions that empower businesses to succeed in their machine learning endeavors.
labelling tool machine learning